2021 will be a year to remember based on technology advances organizations are being forced to implement to keep up with their customer’s demands. Artificial Intelligence (AI) and Machine Learning (ML) budgets are a top priority for companies, and these are accelerating even more as the year continues. In a 2021 study, Algorithmia stated that while there is optimism for the global economy’s direction, there is an urgent need for all businesses to adapt and pivot to new realities. The Covid crisis made clear the profound impact of digitization, especially innovation driven by digital transformation. A 2021 survey found that organizations are making AI and ML a priority across different needs throughout the company in order to reduce costs while improving customer experience. This is the consequence of 2020’s aftermath, forcing companies to be extremely focused on their most important priorities, including AI and ML. Why? Because AI/ML solves the urgency of clear business ROI resulting in the urgency of investing in that industry, making it not a luxury anymore, but a necessity.
At the turn of this decade, there is a surge of no-code AI platforms. More and more businesses are looking to leverage the power of artificial intelligence to build smarter software-based products. But execution becomes an obstacle for many. It’s a challenge for startups to find people with relevant machine learning expertise as the field is always a work in progress, says Anupam Chugh, an independent developer. No-Code AI makes it accessible to all, resulting in organizations worldwide investing in technologies that help them accelerate and democratize data science. Democratizing data science means providing teams with the right tools to solve business problems, operational issues, management, and more with advanced analytical capabilities without the need to possess specialized data science skills. No-Code tools are typically easy-to-use because of their simplicity, like drag and drop visual editors to build ML workflows, a process that otherwise could take weeks to accomplish, depending on the complexity.
Even though building workflows is not as challenging and deploying it, the problems start piling up when they grow in size due to their very complex and sophisticated structure which creates a whole new set of challenges of their own. The largest chunk of work data scientists need to focus on the most is the tasks that come after building and optimizing a model. This means they have 1/3 of the pie assembled, meaning data cleaning, processing, testing, and algorithms in place, but the other side of the pie, which is the most important part because you can’t really serve 1/3 of a pie, is deploying the results of their workflow to their final destination. That could be creating a GET request, exporting a Custom Audience list to Facebook to run a couple of ad campaigns, or sending an RFM model outcome to Intercom to create new product tours with more personalized messaging, and so on.
That right there is the culprit of all the problems companies face when building workflows from Big Data and putting these ML models into production. According to Gartner, 85% of Big Data projects fail, and one of the top 5 factors is the complexity to deploy them.
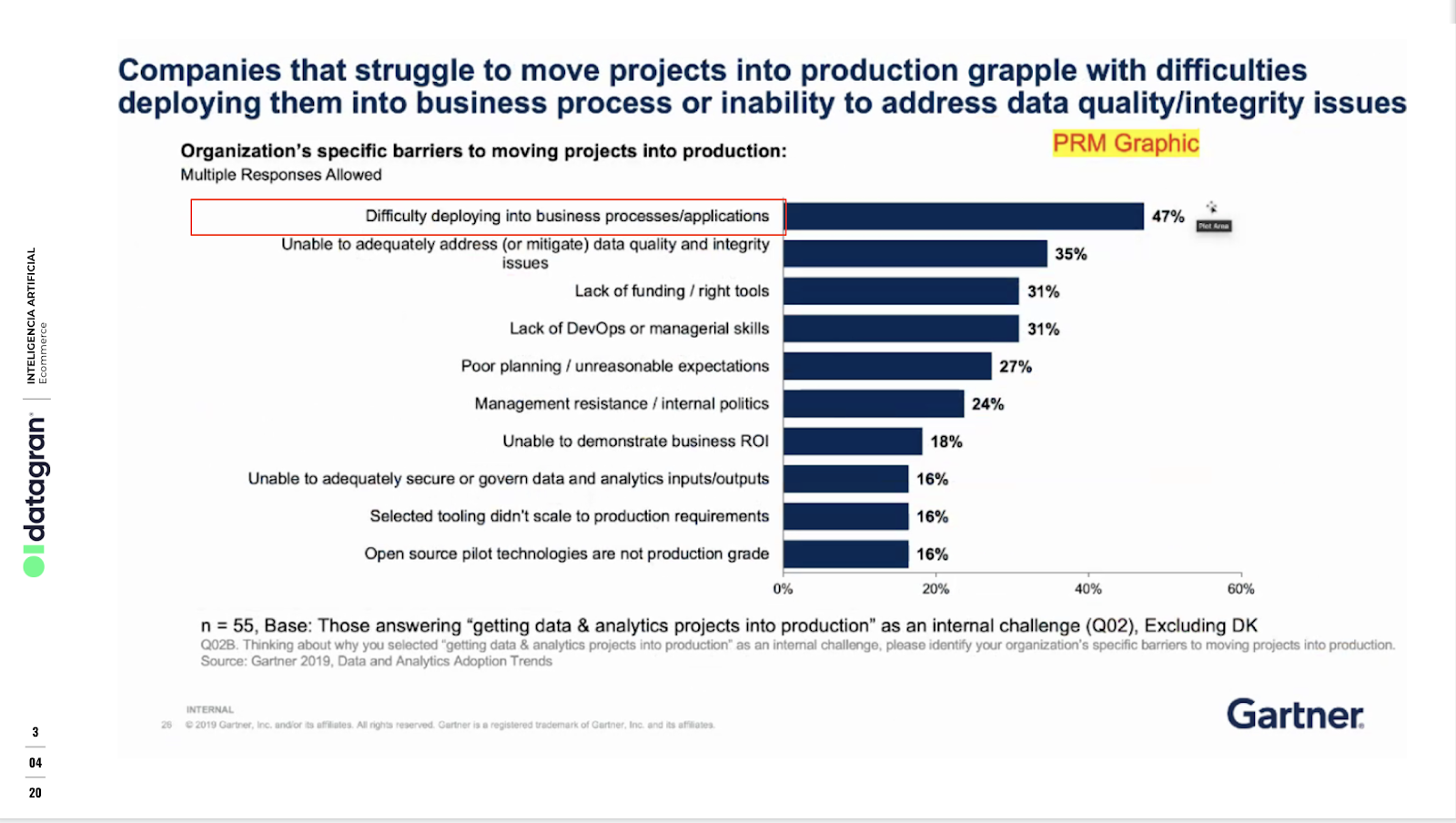

GoDaddy’s Director of Business Analytics stated:
“Our unit has a ton of models but there are just too many pain points to operationalize them”.
This requires data scientists to spend most of their time on model deployment which in many cases, due to the complexities to deploy them, end up stored in some cloud service defeating the purpose of solving some type of business issue. On a 2021 survey, data scientists were asked how much time is being spent on deploying models, which was defined in the question as “prepping trained models and deploying them where they can be consumed by apps or used with other models”. Their data showed that a full 38% of organizations are spending more than 50% of their data scientists’ time on these tasks.
Deploying Machine Learning models require time and specialized skills, as well as emerging/new technologies. In the early days of AI/ML, any organization that wanted to deploy models at scale was essentially required to build and maintain its own system from scratch.
This is a list of technical requirements that a company needed to implement and deploy such models if they decided to build them in-house:
1. Skills or roles:
- A Data Engineer who is in charge of Machine Learning Operations to deploy models.
- A Data Scientist, and a Web Developer to build the APIs needed in order to connect the models’ outputs to business apps.
2. Technology:
- Companies will need to build or use an ELT (Extract Load Transform) tool. This will allow the company to extract data from a specific data source and upload it to a data warehouse or data lake. Some companies doing that are Fivetran or Stitch data. This having in mind some companies choose to build these ELT’s in-house.
- Set up a data warehouse like BigQuery or Databricks
- Setup Spark to be able to run the different algorithms in a distributed way.
- Setup Airflow to build the data pipeline’s workflow.
- Setup the infrastructure scaling when the algorithms are run.
- Once the model’s output is in the Data Warehouse some sort of webhook or API needs to be built in order to send the data to a business application like Salesforce.
2021 still sees many organizations leaning towards the approach above, but this group is typically made out of companies that are running a large number of models. In a recent study, it was found that in organizations with more than 100 models, 60% chose to build and maintain their own systems from scratch, but only 35% made this choice among other organizations. The reason behind this could be because they were considered early adopters and they didn’t have another choice. On the other hand, there are other organizations that are using third-party and No-Code third-party solutions.
Since then, the market has evolved significantly since the early-adopter enterprises started investing in AI and ML. As is evident, organizations more and more have complex infrastructure environments, so third party suppliers like No-Code solutions can be better equipped to support them and save not only time but also a big chunk of money as models scale. In the same 2021 survey, it was found that organizations that buy a third-party solution tend to spend less money on infrastructure and less time on model deployment than organizations that build from scratch.
From meetings held with clients, we always want to know how long is their process to deploy a model from scratch, and typically their answer ranges anywhere from a month to 3 months. This should not happen, and No-code tools are being introduced to solve this problem. Datagran’s platform lets you now build and put ML models into production fast, and without coding. Our clients build complex models that connect multiple types of data sources, include several algorithms like linear regression, clustering, and grouping, and deploy their results to any business application available in our service. Using a No-Code app like Datagran doesn’t mean an organization should change or throw away their current stack, it means the company can introduce a third-party service like Datagran to test and iterate fast and then build Core with their base tools and infrastructure. Test it out by building a simple ML model in Datagran just like Domino’s, Telefonica and Starbucks have.