Predict Future Outcomes, Uncover Risks & Opportunities for your Business with Predictive Analytics
Has your company, for example, done a sales forecast for the next quarter? That means you have been using predictive analytics to know how many sales will be projected for next quarter.
Predictive analytics are increasing in popularity quickly, but what do you need to understand to work hand in hand with your data analysts team to ultimately make the best data-driven decisions?
In this article, we’ll cover the basics of predictive analysis, everything you need to know about what data scientists do so you can feel confident sharing data insights with your teams about the outcomes of predictive analytics.
What is Predictive Analytics?
Predictive analytics is a branch of advanced analytics that makes predictions about future outcomes using historical data combined with statistical modeling, data mining techniques, and machine learning. Companies employ predictive analytics to find patterns in this data to identify risks and opportunities.
Predictive analytics is often associated with big data and data science. Companies today are swimming in data that resides across transactional databases, equipment log files, images, video, sensors, or other data sources. To gain insights from this data, data scientists use deep learning and machine learning algorithms to find patterns and make predictions about future events. These include linear and nonlinear regression, neural networks, support vector machines, and decision trees. Learnings obtained through predictive analytics can then be used further within prescriptive analytics to drive actions based on predictive insights.
We’ve written a few tutorials on regression models. If you feel like jumping right in, use the links below:
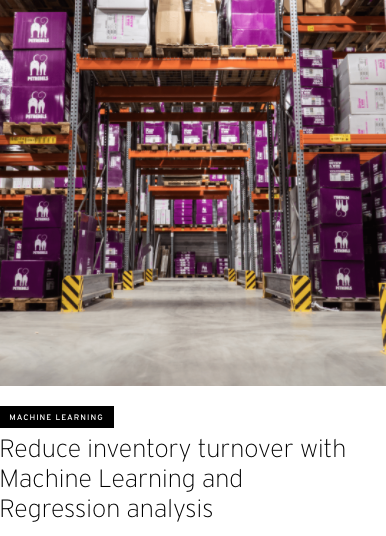
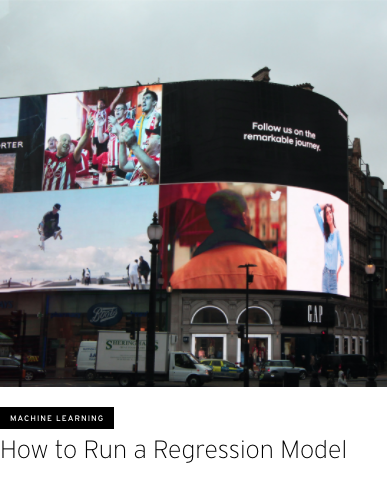
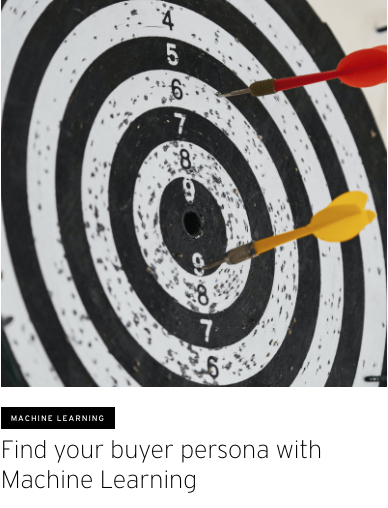
Datagran offers a low-code software tool to help you more easily and quickly build scalable Machine Learning models and put them into production fast.
So why now? Because even though predictive analytics have been around for years, companies are trying to find the best way to make use of their data. And just like electronics, when they’re first launched they’re expensive, but as time goes on, their price drops becoming more accessible to everyone. That means enterprises and mid/large companies aren’t the only ones able to use predictive analytics, SMBs and medium-sized companies are in the perfect moment to make use of them thanks to new tools that are accessible and easy-to-use not only to analysts but to teams with little data science knowledge. Additionally, in today’s tumultuous economic challenges, predictive analytics is more of a need than a want to help solve difficult business problems and discover new opportunities.
How can your business benefit from predictive analytics?
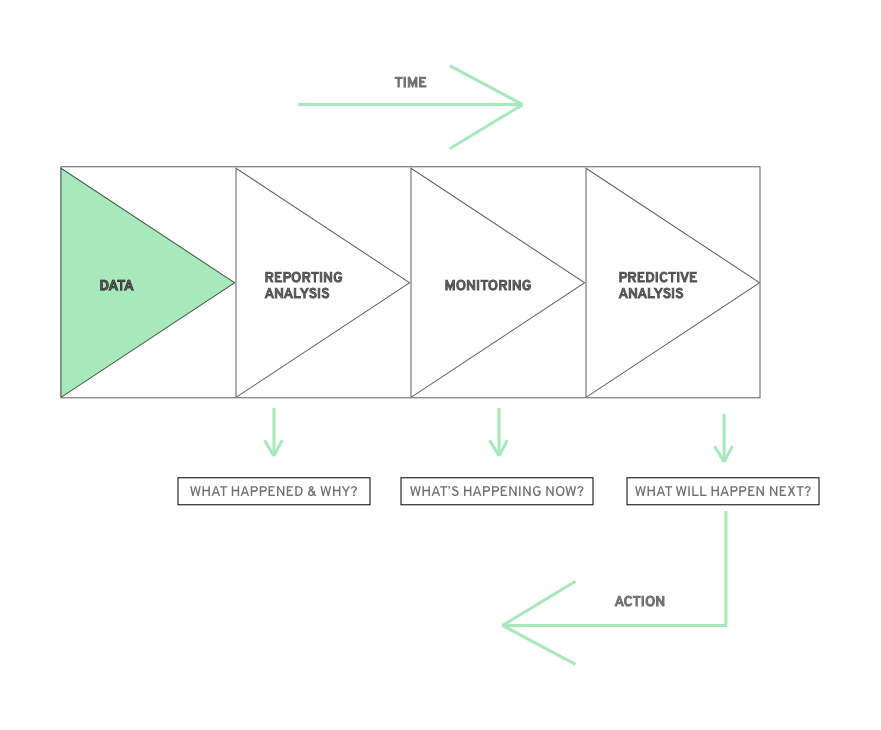
Source Datagran
First, let’s talk about the predictive analytics process.
- Plan
Prior to throwing yourself at the data, you must define your problem, the outcome you want to obtain, expectations, the scope of it, business objectives and the data sources you will pull information from.
- Collect Data
Prepare the data from the various sources– a process called Data Mining. This could mean transactional, behavioral, or other types of data.
- Analysis
Study your data to understand the information your company is currently gathering. With Datagran, the process of analysis is done automatically. You can now inspect, clean transform your data with a simple SQL query or even with a visual editor.
- Test
Data models take a percentage of your data to test and validate assumptions.
- Modeling
Build predictive models about what will happen in the future. Datagran offers a set of algorithms based on Spark or models that are pre-packaged and ready to use with a simple drag and drop.
- Deployment
Deploying predictive models is an issue companies face every single day. In a recent study, Gartner stated that almost 85% of companies fail to put their models in production due to a lack of knowledge, expertise, and tools. Datagran allows companies to put models into production in minutes. Learn how.
- Model monitoring
Get regular updates on the model’s performance to ensure the right information is being used.
Predictive analytics for Risk Management
Now that the process has been laid out, understanding where to apply predictive analytics will be easier to understand.
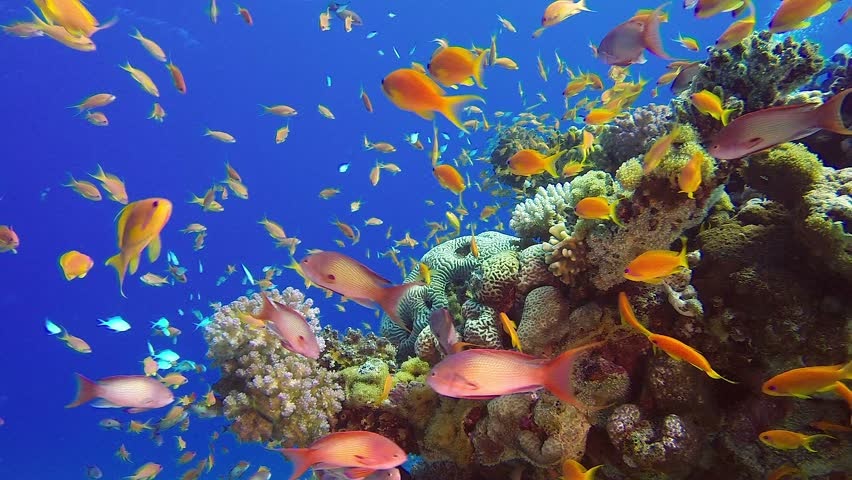
Source: Google
Use Case: EachMile needed to understand and predict the health of harvested fish to consequently predict the availability in the supply chain.
For EachMile, a non-profit organization focused on transforming the global seafood and agriculture supply chains, using predictive analytics was a need they needed to incorporate into their business. EachMile needed to understand and predict the health of harvested fish to consequently predict the availability in the supply chain.
The team at Datagran, guided them through the predictive analysis they needed to build to accomplish their goal. And following the same structure mentioned in the step-by-step above, EachMile centralized weather data, temperature, and quality of water, acidity, among other variables via IOT sensors in order to predict fish health. And to predict the volume in the supply chain. Additionally, they needed to create awareness among fisheries with the goal of increasing the volume of healthy fish in the oceans by sharing the model’s predictions of how the water should be maintained for optimal fish health.
The process included several predictive models using grouping and classification algorithms that provided the output they expected. Lastly, they took the model’s output and sent it across multiple fisheries via Datagran’s REST API Action in minutes so a combined effort to save fish health was done throughout different communities.
Optimizing marketing campaigns
Predictive analytics are used to determine customer behavior, as well as take advantage of cross-selling. Predictive models help businesses attract, retain and grow their best and worst customers.
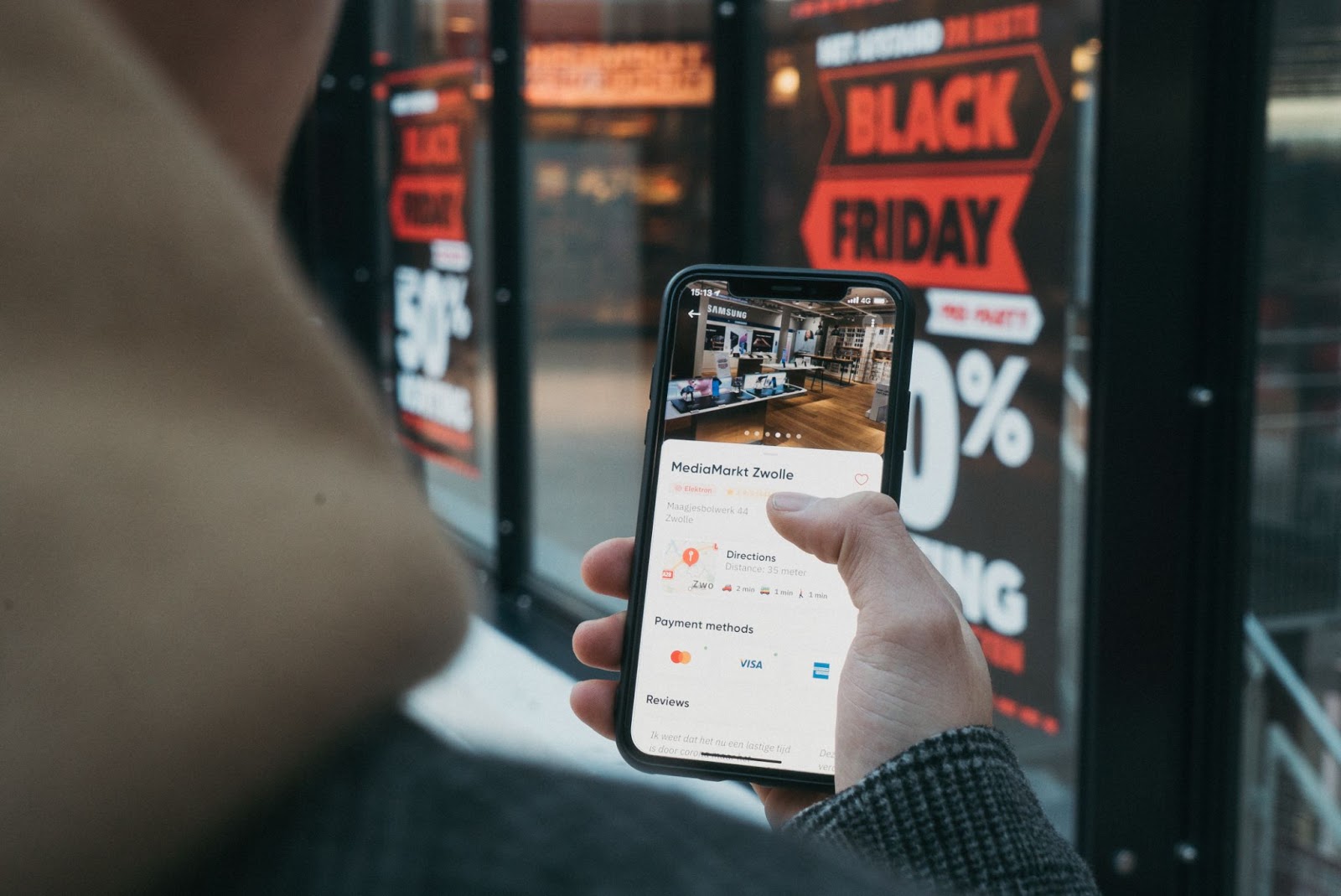
Source: Unsplash
Use Case: I want to know which products will be selling next month.
Almost everyone who runs an e-commerce business has issues guessing inventory numbers. Trends shift rapidly, and customers make decisions even faster. With predictive analytics, our customers have been able to run Recommended Product models to predict which products will be sold the most, to which customers with additional recommendations of products some customers may have not known about. For a multi-national coffee company, this came in handy thanks to them being able to run predictive models with their own data. Targeting customers who only purchased a black coffee and a pastry every morning, the model was able to predict based on historic shopping behavior, that they would be the perfect set of customers to target with their other type of pastry which increased revenue by a said percentage. The company used Datagran’s REST API tool to send personalized promotional content to their segmented customers, increasing profits and accomplishing their goals.
Other uses:
- Financial Institutions
- Cross-Selling
- Fraud detection
- Health care
How do predictive models work?
Predictive models take results from your data to develop or train a model that can be used to predict things happening for different or new data. The outcome is provided in predictions that are probabilities of a variable, such as revenue.
There are two types of models:
- Classification models
A classification model draws conclusions from observed values. Given one or more inputs a classification model will try to predict the value of one or more outcomes. Outcomes are labels that can be applied to a dataset. For example, when filtering emails “spam” or “not spam”, or when looking at transaction data, “fraudulent”, or “authorized”
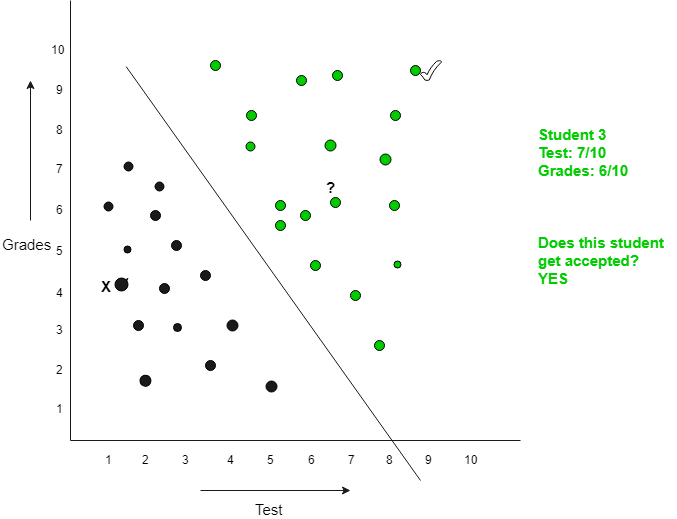
Learn more about classification models here.
- Regression models
Regression is a statistical relationship between two or more variables where a change in the independent variable is associated with a change in the dependent variable. There are various types of regression and they are valuable to solve key business problems across key industries like healthcare, financial services, retail among others.
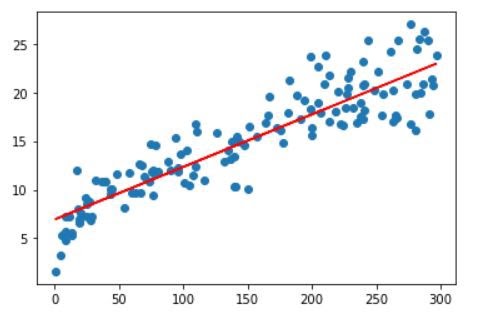
Learn more about regression models in this post.
Datagran offers both algorithms to build scalable models with little to no code. With an easy-to-use user interface, your teams can create regression, classification, RFM, recommended products, clustering, among many other models with multiple data sources, and send them into production in minutes or days instead of months which is the average time a model is said to take.
By building predictive models, organizations can tap into key data that can draw valuable insights in no time. And even though many companies have the right technology, most lack the organizational capacity to take full advantage of predictive analytics. In addition, many organizational processes aren’t built to make use of analytics and make it a competitive advantage. High-performing organizations take advantage of the power of analytics by funneling their efforts in four areas: focus, adopt, adapt, and activate. These companies have embraced a new paradigm that promotes agility, fast execution, and lasting organizational change.